The ROI of AI – From Buzzword to Impact
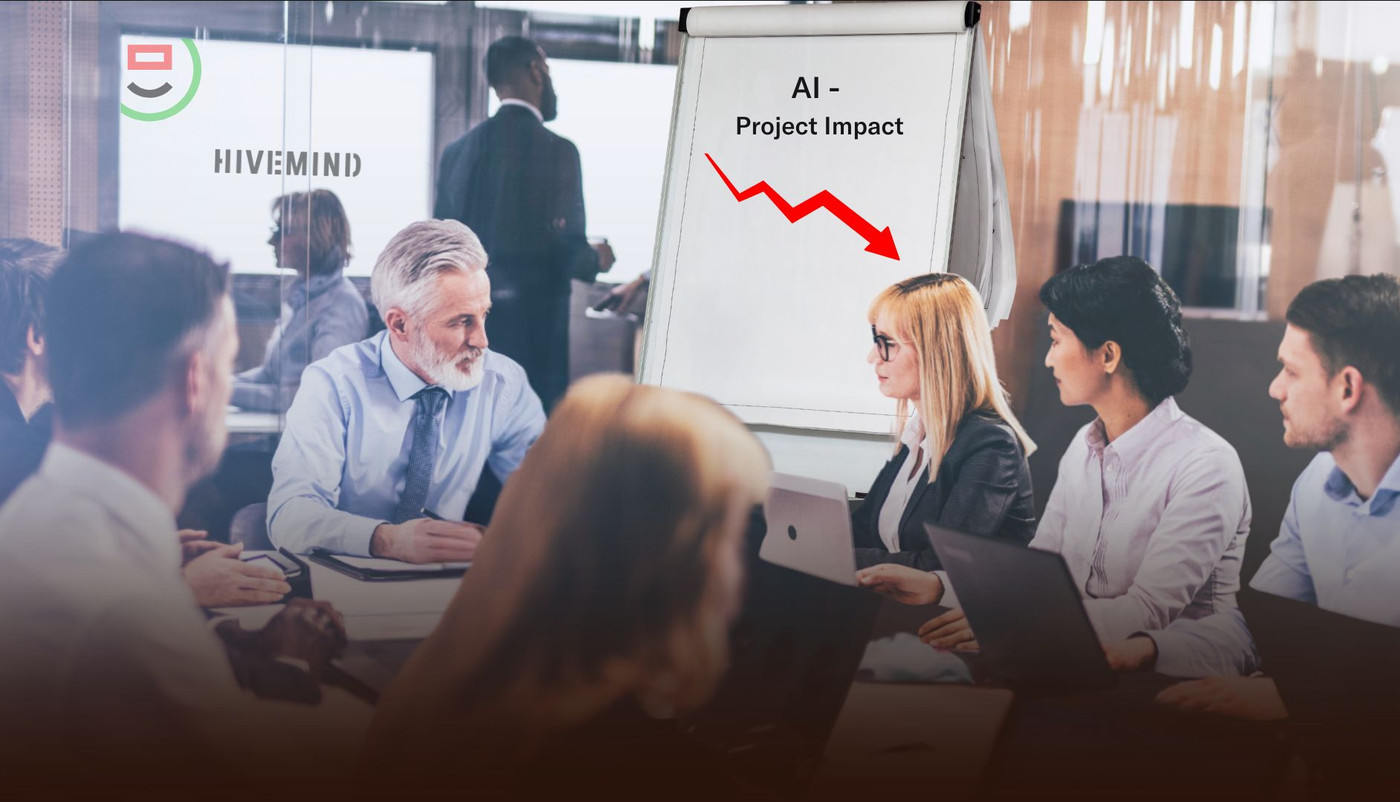
Artificial intelligence (AI) is being hailed as the key technology of our time, but there's often a gap between the hype and the real business value. Many organisations ask themselves: How can AI move beyond being just a buzzword and actually deliver a measurable return on investment (ROI)? In this article, we explore how mid-sized and large enterprises can evaluate the ROI of AI initiatives, why so many AI projects get stuck in the buzzword trap, and how Hivemind Technologies applies a structured methodology and effective tooling to transform AI from a mere concept into a value driver. We will also examine real-world use cases from different industry sectors.
Measuring AI ROI: Quantifying the Value Clearly
Before investing in AI, it is essential to define what success looks like. ROI, or return on invested capital, can only be measured if there are established objectives and metrics. This process involves establishing tangible key performance indicators (KPIs), such as:
Financial KPIs: Increases in revenue (e.g., via personalized offers) or cost savings (e.g., through automation).
Operational KPIs: Enhanced productivity (like time savings from automation bots) or fewer errors (for example, through better fraud detection).
Strategic KPIs: Faster innovation cycles, improved competitive positioning, or higher customer satisfaction.
This multidimensional approach captures the full value of AI, including direct profits as well as efficiency gains and future-proofing. It is also important to consider the time horizon: some AI initiatives, such as process automation, deliver quick wins, whereas others take longer to mature and may only yield returns in the medium term. By establishing clearly defined, relevant KPIs from the outset and balancing short-term wins with long-term goals, businesses can create a firm foundation for measuring their AI initiatives' ROI. This ensures that AI investments remain closely aligned with corporate strategy and that the resulting gains can be clearly demonstrated afterwards.
The Buzzword Trap: Why Many AI Projects Fail
Despite large investments in AI, widespread success remains elusive. Studies paint a sobering picture: only about 8% of companies generate significant financial benefits from AI, while over 80% of AI projects fail to meet their goals. This sharp discrepancy highlights the peril of the buzzword trap: implementing AI simply because “everyone else does,” without a solid business rationale.
Typical reasons for AI initiatives to falter include lack of clear targets, insufficient focus on tangible value, and unrealistic expectations. Projects often begin with no precise use-case or success criteria—in one survey, only 34% of data scientists reported having clearly defined project targets from the outset. As a result, pilot projects may look technologically exciting but never transition to production because their value proposition is murky. Meanwhile, AI hype can lead to overblown expectations among senior leadership and end users. AI is often presented as a silver bullet, and when the reality sets in—such as lengthy data preparation or the need for significant process adjustments—disappointment typically follows.
Another facet of the buzzword trap is “AI-washing”—slapping the AI label onto projects to appear cutting-edge, even when basic analytics would suffice. This distorts perception and distracts from genuine business objectives. In the end, budgets get spent without measurable returns, fueling internal skepticism about AI. The best way to avoid this is to view AI through an ROI lens right from the start: Which core business metric is this AI solution expected to enhance, and how exactly will data and models deliver that improvement? That’s how AI can transcend buzzwords to become a true business enabler.
A Structured ROI Methodology: How Hivemind Technologies Provides Direction
How can organizations escape this cycle of hype and disillusionment? The answer lies in a methodical approach that anchors AI initiatives firmly to value generation. This is precisely the focus at Hivemind Technologies: leveraging a proven ROI methodology and robust tooling, we support businesses from ideation to production—always with business impact in mind.
One key to success is treating AI initiatives as business projects, not isolated technology experiments. That’s why Hivemind first helps clients pick the right use cases: which specific pain points can AI realistically solve? Together, we quantify opportunities before any coding begins. Cost-benefit analyses and proof-of-value testing lead the way, ensuring that each idea is backed by a viable business case.
This approach is grounded in a phase model that tracks project performance at every stage:
Define clear goals: Right from the start, measurable success criteria are established (e.g., reducing maintenance costs by 10% or cutting lead times by 20%). These KPIs guide the project.
Assess data and feasibility: We evaluate data availability and quality, and gauge the technical effort required. This ensures that projects can realistically achieve their projected ROI.
Iterative development with an MVP: Rather than going for a “big bang,” Hivemind follows an agile approach. We work in short cycles—developing a Minimum Viable Product (MVP), then enhancing it. We measure the relevant KPIs even in the MVP phase to validate early on whether the project is on track.
ROI tracking and optimization: Through smart tooling, we continuously monitor the performance of the AI solution. Dashboards show in real time whether the expected gains or efficiency improvements are materializing. If necessary, we refine the model or adjust the use case—data-driven feedback is a core component.
Scaling and rollout: When the solution proves its worth at a small scale, Hivemind assists in rolling it out across other areas or sites. In parallel, we help the client build internal expertise so that AI becomes a permanent part of the organizational fabric.
This structured approach delivers transparency and trust: at each phase, stakeholders can see how the AI solution is adding value. That makes sure AI projects live up to expectations and earn acceptance throughout the organization. While thought leadership alone doesn’t guarantee success, combining it with clear methodology and proper tooling makes the path from idea to impact tangible. As noted in a recent analysis, focusing on carefully chosen use cases with relevant, quantifiable metrics is critical for unlocking AI’s full potential. That’s exactly Hivemind Technologies’ mission: providing clarity in the AI jungle and ensuring that promising pilots translate into measurable successes.
Real-World AI Use Cases: Where ROI Is Already Showing
Methodology and frameworks are important, but nothing is more convincing than hands-on examples. Across many industries, AI has already proven its ability to drive bottom-line results. Below are several use cases from finance, manufacturing, e-mobility, energy, and green tech, illustrating how AI is delivering measurable value:
Predictive Maintenance in Manufacturing: Companies leverage AI to predict maintenance needs before machinery breaks down. Sensor and machine data are analyzed to spot early warning signs of equipment failure. This leads to fewer unplanned outages and more efficient maintenance schedules. According to a Deloitte study, predictive maintenance solutions can lower maintenance costs by up to 25% and slash unplanned downtime by up to 50%, a significant ROI resulting from better machine availability and longer equipment lifespans.
AI-Based Credit Scoring in Finance: Banks and financial institutions are increasingly turning to AI to support credit decision-making and improve efficiency. Modern models can analyze hundreds of variables—such as payment behavior, transaction histories, and relevant external data sources—in a fraction of the time it used to take. Rather than fully replacing human judgment, these systems work best as decision support tools that significantly reduce the manual effort required to evaluate applications. A human-in-the-loop approach helps ensure fairness, transparency, and accountability—especially in sensitive contexts where financial prospects are at stake. Over time, with careful training, robust oversight, and in less sensitive application areas, even real-time decisioning can become feasible and responsible—offering both speed and trustworthiness.
Load Forecasting in Energy: Power utilities must accurately balance supply and demand, especially with the growing share of renewables. AI-powered load forecasting uses historical consumption data, weather patterns, and other signals to predict electricity needs more precisely. Greater accuracy translates directly into cost savings: utilities can match production or procurement closely to demand and minimize costly overcapacity. At the same time, grid stability improves. As IBM has noted, high-quality load forecasting can help utilities avoid losses from under- or overproduction and significantly boost system efficiency. For providers, that means lower costs, higher returns, and more stable networks—a clear win from AI.
Sustainability Analytics in E-Mobility and Green Tech: In green technology and e-mobility, data analytics plays a central role in achieving environmental objectives. AI can process massive datasets on emissions, energy usage, and resource lifecycles, helping companies assess and enhance their sustainability performance. A prime example is managing electric vehicle fleets: AI models can predict battery lifespans and identify optimal charging schedules to minimize degradation. They can also track real-time CO₂ savings from e-mobility. In green manufacturing, AI-based supply chain and lifecycle analyses help uncover hidden inefficiencies or resource waste. The ROI can be monetary—e.g., by lowering energy use or extending product life cycles—and it can also include regulatory compliance and meeting ESG criteria, which can open up long-term market advantages. Not least, operating more sustainably improves brand equity and resonates with customers and investors.
Conclusion: Moving Beyond Buzzwords to True Impact
AI doesn’t have to be an end in itself—done right, it becomes a powerful value driver. IT leaders should ask for each AI initiative: How does this solve a core business challenge? When the answers are crystal clear, implementation can begin in a measured way, and success is tracked continuously, turning AI from hype into real business impact. The success formula is clear: substance over hype.
To move from experimentation to measurable ROI, start by looking at your existing standard operating procedures (SOPs). Focus on the steps that are time-consuming, error-prone, or dependent on unstructured data—like emails, reports, meeting notes, images, or audio. These are often overlooked areas where AI can make a real difference. With today’s advanced language technologies—LLMs, NLP, speech-to-text, text-to-speech, and image-to-text—you can convert this messy input into structured, actionable information. Even in a multimodal world, the common thread is still text: understanding it, generating it, and integrating it into your workflows. By targeting these high-friction points—especially ones that were too complex to automate in the past—you open the door to new levels of efficiency and impact.
Hivemind Technologies sees itself as a trusted advisor in this space. With extensive experience, deep industry expertise, and a structured ROI methodology, we help companies separate the wheat from the chaff. From initial brainstorming through pilot projects and enterprise-wide rollout, we make sure AI innovations yield sustainable benefits—measurable, transparent, and aligned with organizational strategy. This is how to transform AI’s remarkable potential into tangible results. AI ROI then becomes more than just a headline: it’s realized in practice, turning the buzzword into genuine impact—and investment into real returns.